Is the Requirement for Point Cloud Classification Dead?
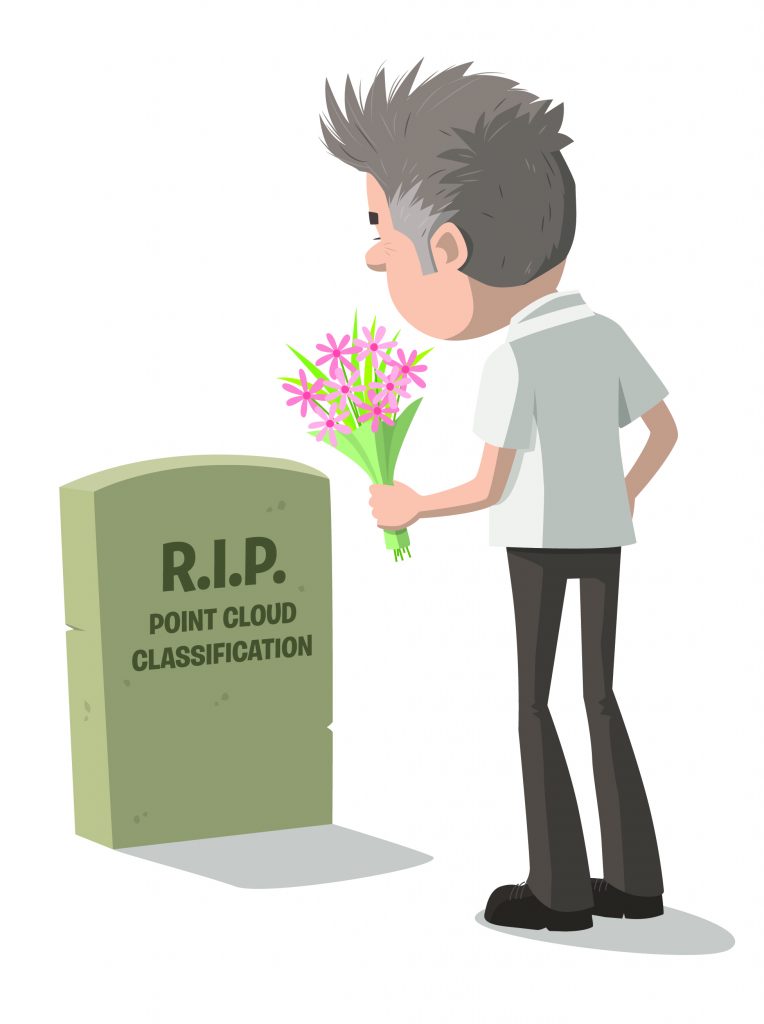
Mobile LiDAR system (MLS) point cloud delivery requirements often call for the initial “classification” of the point cloud data; typically identified as Ground, Low Vegetation, Medium Vegetation and High Vegetation. In recent years, especially in the North American market, such classification requirements for MLS data have now been largely discarded. In this discussion Professor Topo examines the origins of the classification requirement for MLS data and demonstrate how modern feature extraction techniques make this time consuming classification step not only unnecessary, but unproductive as well.
Origins of the Classification Requirement
We first note commercial airborne LiDAR systems (ALS) preceded MLS platforms by at least two decades. The spatial density of the point cloud data produced by these ALSs was typically about one point per square meter. Thus the data was basically used for wide area terrain mapping applications of about 1m+ accuracy.
One of the first software application challenges for extracting topography models from this data was to effectively “clean it up” by identifying and isolating points attributed to the ground and removing those from vegetation; hence the requirement and naming convention for classified data.
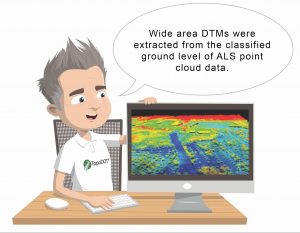
3D digital terrain meshes (DTM) were then automatically extracted from the ground classified point cloud. Now it should be pointed out that these DTMs were basic terrain models over wide areas. This data and the extracted DTM found many practical uses for large scale planning, power transmission line survey, forestry, hydrology among others.
However the ALS data typically did “not” support feature extraction of sufficient accuracy and precision to meet traditional corridor survey requirements. Thus the format and structure of the extracted DTMs proved inadequate to meet the requirements of downstream planning, engineering and construction operations.
The Advent of MLS Data
The advent of terrestrial MLS changed point data characteristics dramatically. Data acquired along a roadway (or railway) corridor from such relatively short range increased the spatial density of the point cloud by several orders of magnitude. This high density data facilitated identification and extraction of topographic features such as break line vectors, signs, pavement markings, utility covers, etc. Close proximity to control survey reference targets facilitated more accurate registration of data.
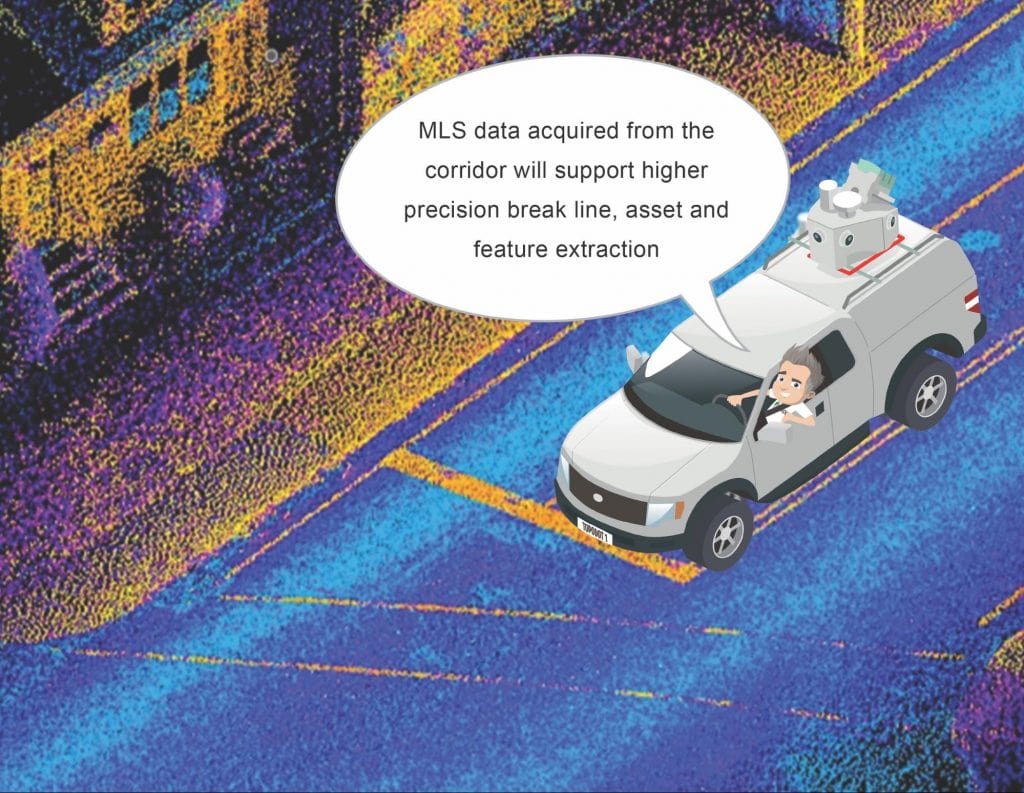
MLS data characteristics showed the potential to support the extraction of topography models meeting design, engineering and construction requirements. However, these topographic models require significantly more metadata and precision than the wide area DTMs. Specific break lines, features and assets must be clearly identified within established CAD formats and extracted to very high levels of precision.
Earlier attempts to extract topographic models employed the same legacy software applied to ALS data for generating wide area DTMs. It became immediately evident that the surface mesh extraction tools were incapable of identifying and extracting features with any high level of precision. Furthermore the classification of data into traditional Ground and Vegetation levels made little to no contribution to the data extraction process.
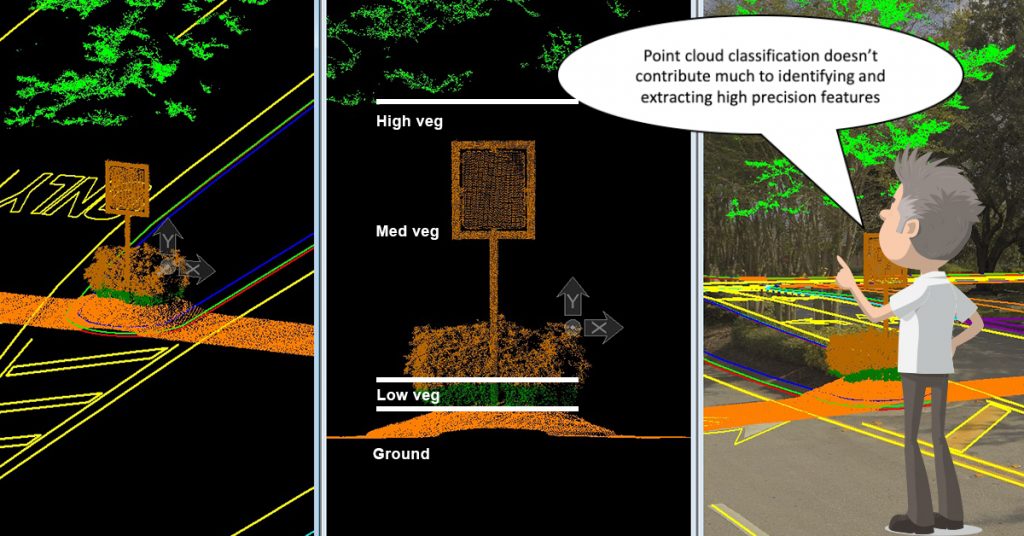
Not only were important break line features unidentified, but the mesh represented topography breaks with “less” accuracy than the original point cloud data. Thus the legacy software process requiring data classification proved mostly inadequate in meeting the format, structure and accuracy requirements of traditional corridor topographic models.
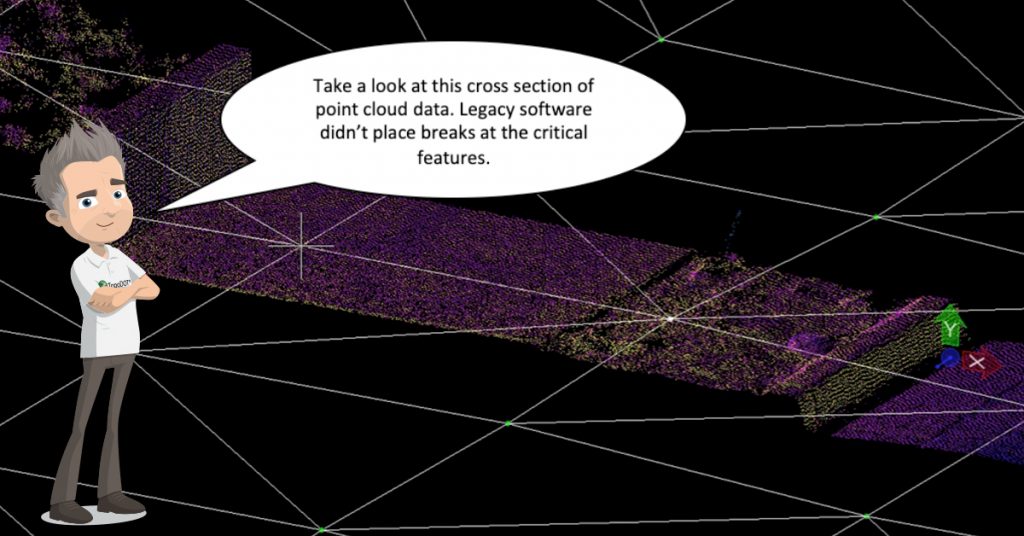
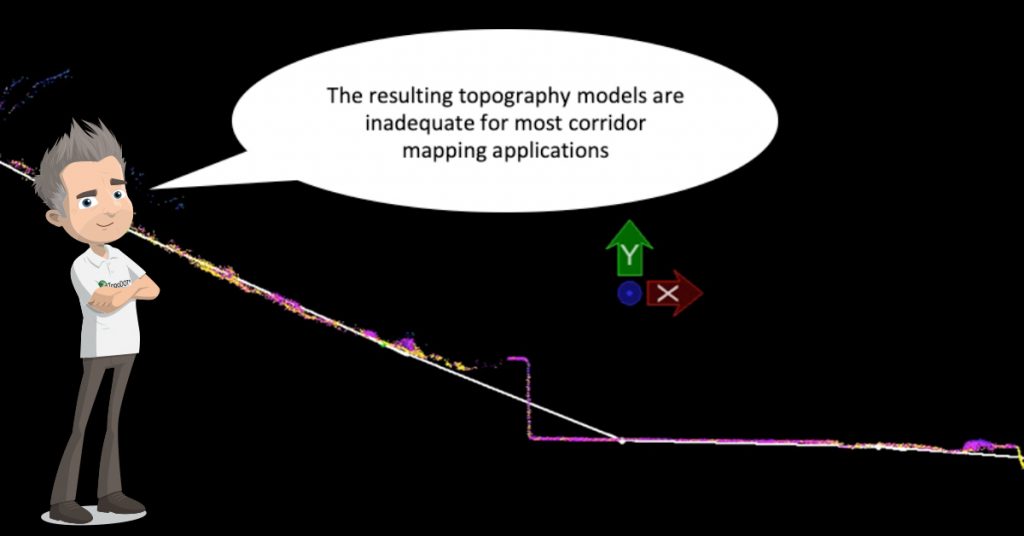
TopoDOT® Extracts High Quality Models Directly from Unclassified Data
The limitations of the legacy ALS software solutions made it clear a new approach to topography and model extraction was necessary to meet the requirements of transportation corridor applications. Team TopoDOT proceeded to meet this challenge by first recognizing that a topography model was comprised of many different features and that each feature had its’ own unique structure and characteristics.
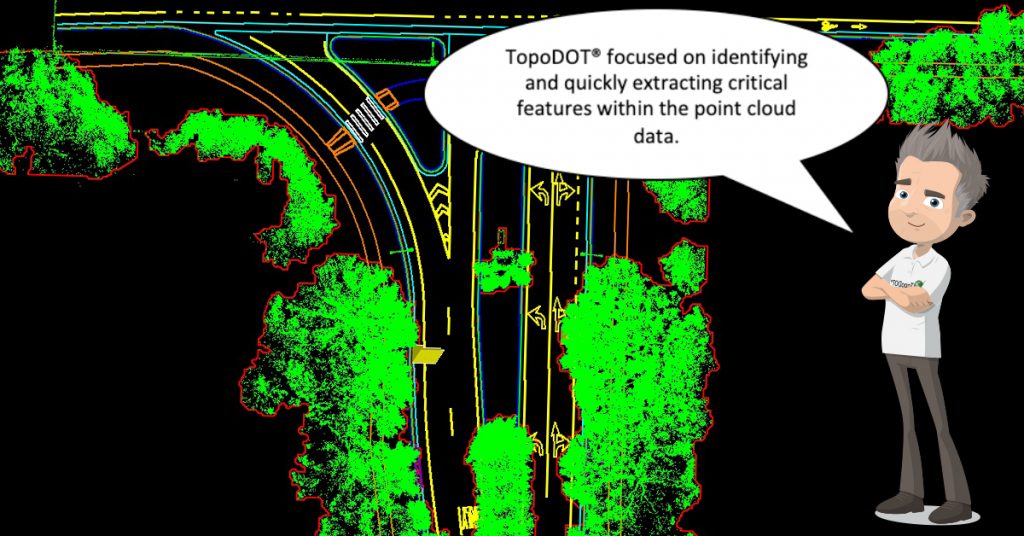
What about the data noise?
Team TopoDOT realized that an advantage of exploiting the unique structure of each feature for extraction is that the data not associated with the feature is more easily identified. Thus TopoDOT has implemented filters within each feature extraction tool designed to ignore data not associated with the specific feature being extracted. This has profound operational advantages, primarily eliminating the need for data classification or clean-up!
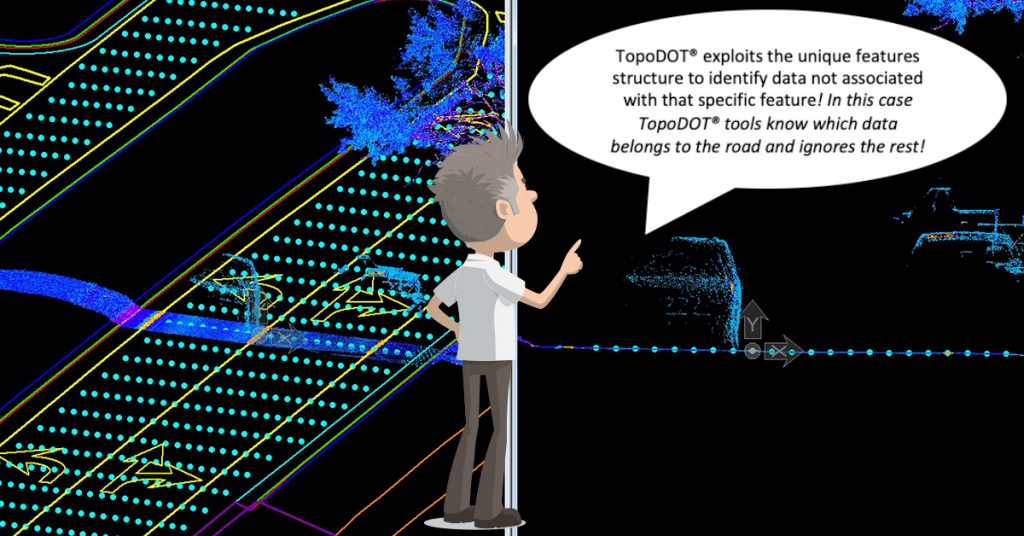
To extract the topography, team TopoDOT abandoned the “one-algorithm-fits-all” approach to surface model extraction. Today TopoDOT tools are designed to exploit the inherent data structure of a specific topographic feature. So for example, the Break Line Extraction Tool would be used to extract curbs, the Road Extraction Tool to extract the road surface, the Elevation Grid Tool to extract bare earth surfaces, and the Asset Extraction Tool identifies and extracts signs, poles, and other assets.
These features are then integrated into the construction of a complete topography model. This is a highly automated process resident within most CAD software applications. Typically break lines, elevation points and other features extracted within TopoDOT are identified to the DTM engine. The engine then constructs the surface model from these features. As illustrated in the following, the resulting DTM maintains the precision and accuracy of the original point cloud while meeting the format requirements necessary to meet downstream design, engineering and construction operations.
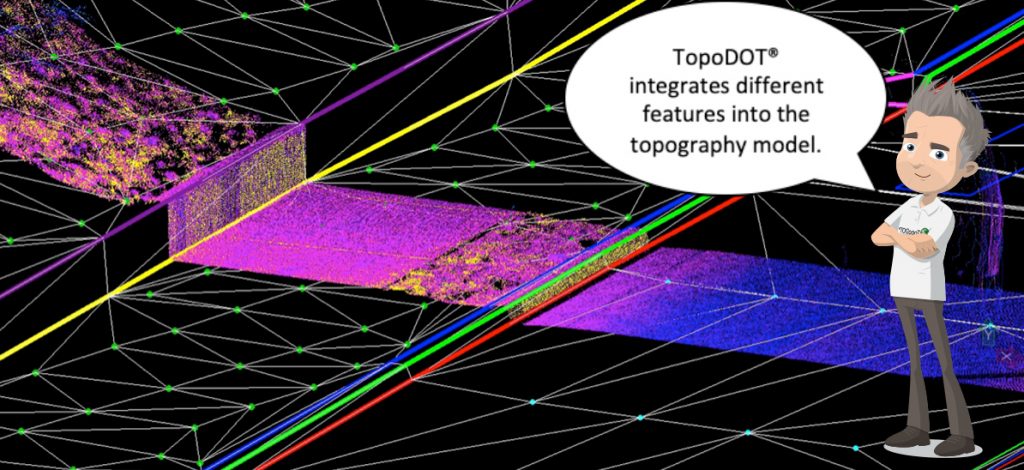
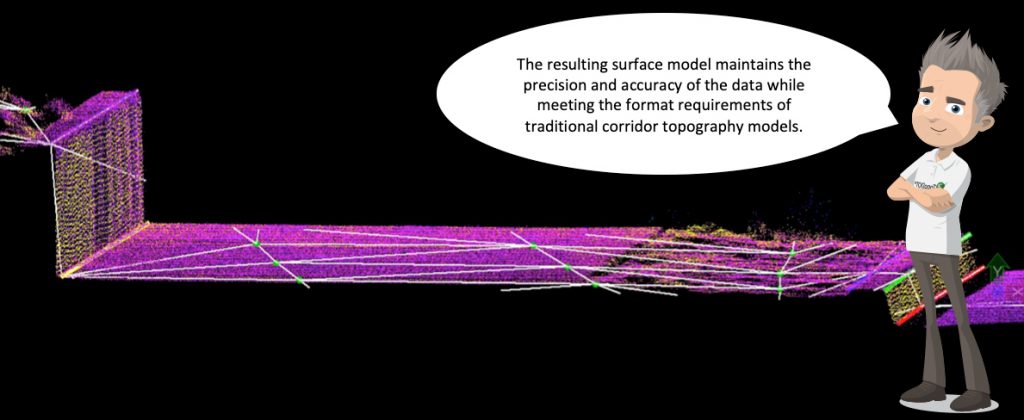
Is Classification Really Dead?
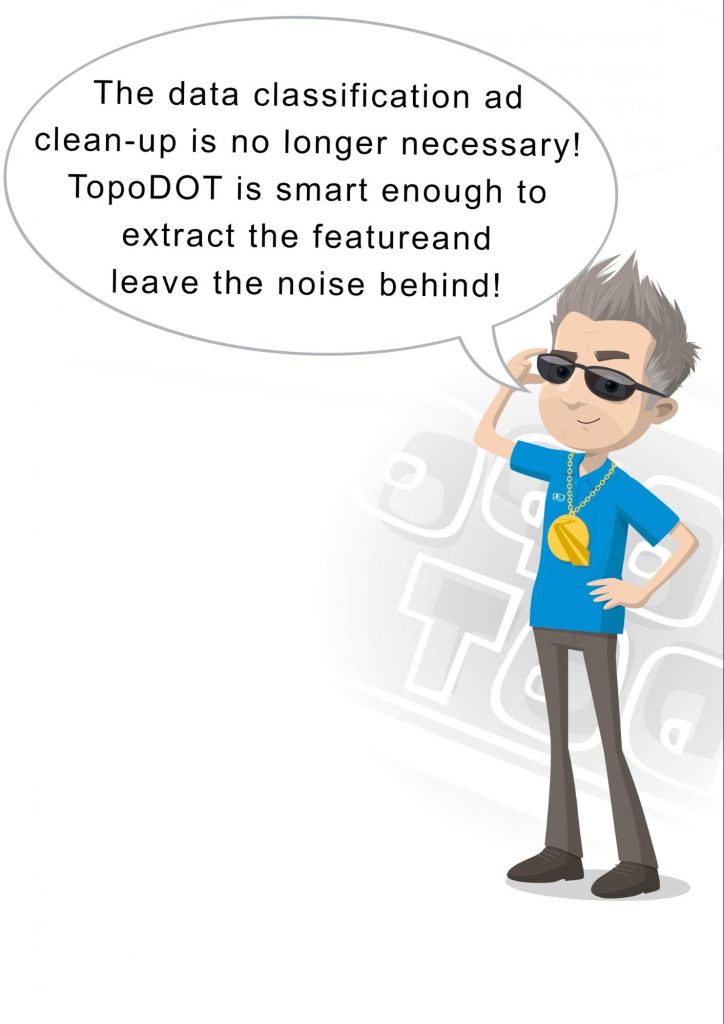
TopoDOT does offer traditional data classification and classified data import capability. TopoDOT has also expanded data classification in support of operations within the TopoDOT Analysis tool suite. Classification has proven an effective tool in the areas of measurement extraction, monitoring of movement, verification of design and vehicle clash simulation within the point cloud. Therefore, classification is not dead! Rather it is largely confined to operations in which the classified data contributes to a specific analysis objective within localized areas of the point cloud.
The Bottom Line
Long and tedious data classification/clean-up operations are no longer necessary to support topography extraction! TopoDOT extraction tools effectively filter out extraneous data in parallel with extraction of the feature itself. The result is a faster process and a 3D topography model exceeding the requirements of downstream design, engineering and construction operations.
Did you find this TechNote informative? Visit https://new.certainty3d.com/blog/technotes/ for a collection of TechNotes describing the practical use of point cloud data in real world applications.
Would you like to learn more about TopoDOT? Please visit https://new.certainty3d.com/ , request a demonstration at https://new.certainty3d.com/contact/demo, or contact us directly at: 001 407 248 0160 / info@topodot.com
2 Comments
Ryan C. Swingley · April 11, 2019 at 3:35 PM
I agree that detailed classification is unnecessary with TopoDOT’s tools. I still run a simple ground, low, medium and high vegetation classification for visual inspection purposes. Great read!
Ted Knaak · April 11, 2019 at 4:00 PM
@ryan swingley I agree and realize a simple classification can be a convenience. In fact I state in the article that classification can be very useful. But what we’re trying to address is the hard requirements that we still see mostly in European transportation agencies for pre-classification of data. This necessitates that entire projects be classified instead of as you mention, classifying some local areas for convenience during the extraction process. This classification requirement tends to also focus all parties on software capabilities that really are no longer relevant. For example, when evaluating software, performance of the “ground” classification tool is intensely compared when it really doesn’t matter! In summary, classification is now more a secondary convenience, and no longer a primary requirement.